GPU Prices
October 3, 2024
•
Johan Backman
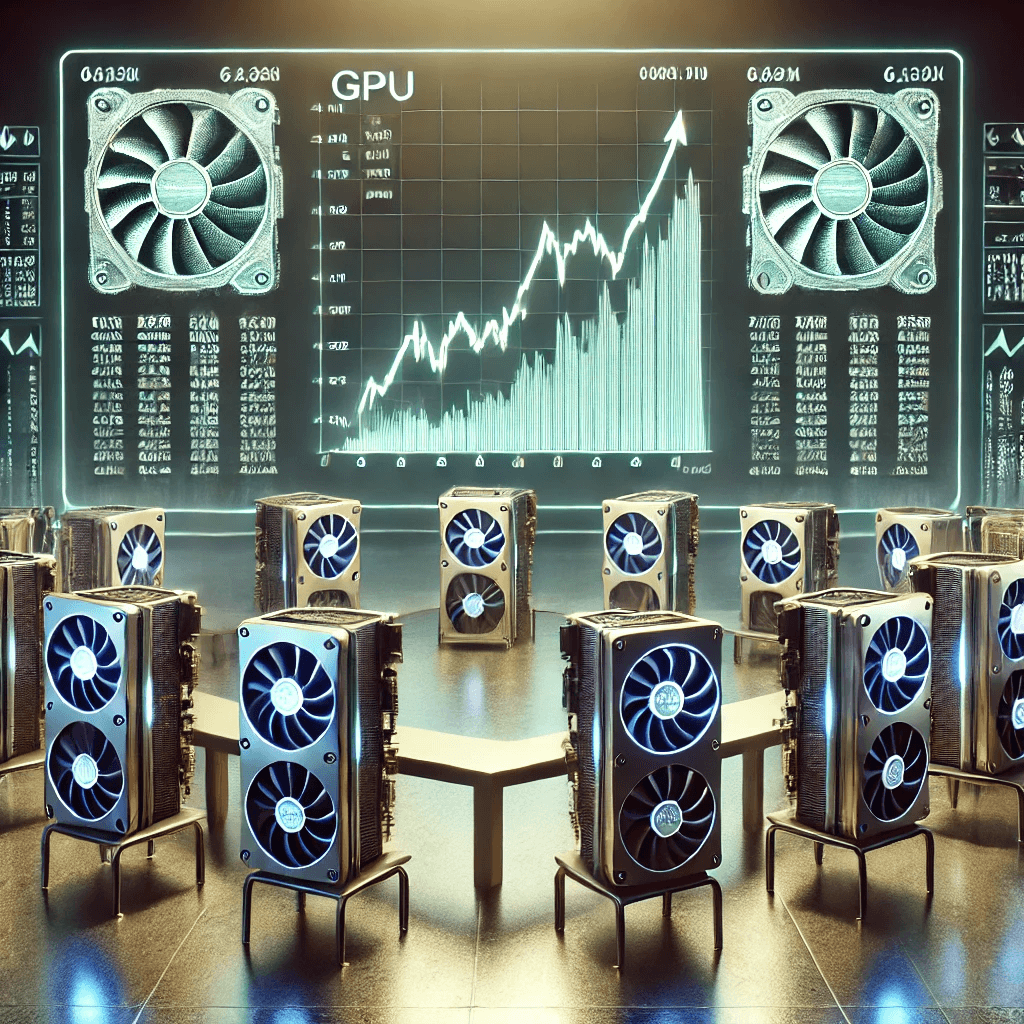
In this article, we're going to look at pricing across various providers and compare them to Trainwave. Everyone wants a better price and you guessed it, GPUs are becoming a commodity. Sure you can go to AWS and pay premium price, but did you ask yourself why?
Why pay AWS or any other major cloud provider?
You could be paying for the convenience, the support, or the ease of use. But what if you don't need that? What if you just need raw compute power? That's where Trainwave comes in. We offer the same GPUs and similar service as the major cloud providers, but at a fraction of the cost. Before we get all excited about what we do, let's actually list out the pros of the major cloud providers:
Pros:
- Configurability: Lots of options for you to pick and choose from
- Support: Premium support if anything goes wrong
- Network speeds: The beefier the machine the better the network speeds
Cons:
- Complexity: Don't forget to configure IAM roles, security groups, and VPCs before you get started!
- Price: Literally paying the top dollar for the hardware
- Vendor lock-in: Once you're in, it's hard to get out
The real question is are you ready to pay top of market for those pros? If not, keep reading.
Why consider an alternative?
The uptime, the configurability, the premium support, and the network speeds are all great, but what if you don't need that? What if you just need raw compute power? Oftentimes machine learning workloads are geared towards compute power more than uptime. AWS is great when it comes to hosting your online service, but do you need to pay that top dollar for some training job?
We don't think so, which is how trainwave was born. We realized we could run our ML workloads anywhere as long as there were good GPUs to run on.
The numbers
Let's look at a couple of examples to get an understanding what you'd be paying at the different clouds.
The table below outlines the cost of using each clouds ML service together with the GPUs.
GPU type | Trainwave | AWS | GCP | Azure | Markup |
---|---|---|---|---|---|
8x H100 | $38.99 | $113.07 | $101.01 | N/A ** | 2.6-2.9x |
8x A100 (80GB) | $23.86 | ~$47* | N/A | N/A ** | 2x |
8x A100 (40GB) | N/A | $37.69 | $33.80 | $27.20 | N/A |
8x V100 | $3.56 | $28.15 | N/A | $24.48 *** | 6.9-7.9x |
* Some GPUs are in preview and pricing is not publicly available. Pricing is estimated based on EC2 => Sagemaker markup
** Azure does not seem to have a similar offering at this time
*** Azure does not have 8x only 4x. Price is estimated
We also found that both AWS and GCP marks up their hardware by 15% to use their ML services (Sagemaker, Vertex).
$/TFLOPs
To make an even better apples to apples comparison, we look at FLOAT16 Operations/s on the GPUs.
The below table augments the previous table with TFLOP numbers and $/TFLOP
GPU type | TFLOPS | Trainwave ($/TFLOPs) | AWS ($/TFLOPs) | GCP ($/TFLOPs) | Azure ($/TFLOPs) |
---|---|---|---|---|---|
8x H100 | 1979 | $0.0197 | $0.05713 | $0.0510 | N/A |
8x A100 (80GB) | 312 | $0.0765 | $0.15064 | N/A | N/A |
8x A100 (40GB) | 312 | $0.0765 | $0.1208 | $0.1083 | $0.08718 |
8x V100 | 14 | $0.2542 | $2.01 | N/A | $1.748 |
As you can see it's important to understand the requirements of your model and match the GPU, you could be paying $2/TFLOP instead of $2c/TFLOP, which is 100x more expensive!
Conclusions
NVIDIA is going to the moon, major clouds are profiting. But what about you? Are you getting the best bang for buck? We hope this article has shed some light on the pricing of GPUs across the major cloud providers. If you're looking to save some money, Trainwave is here to help. We offer the same GPUs and similar service as the major cloud providers, but at a fraction of the cost.